Remove cell cycle from perturbed mESC¶
Here we use the mESC dataset with 600 cell cycle genes and 1000 other gene expressions doubled for 40% cells. For simplicity we have converted the dataset into TPM.
Import necessary packages¶
[1]:
%load_ext autoreload
%autoreload 1
[2]:
import sys
import pandas as pd
import numpy as np
import pickle as pkl
import sklearn as skl
import sklearn.preprocessing
import matplotlib as mpl
import matplotlib.pyplot as plt
Warning information from TensorFlow may occur. It doesn’t matter.
[3]:
import tensorflow as tf
tf.set_random_seed(0)
import cyclum
from cyclum import writer
/home/shaoheng/.conda/envs/tensorflow-gpu/lib/python3.6/site-packages/h5py/__init__.py:36: FutureWarning: Conversion of the second argument of issubdtype from `float` to `np.floating` is deprecated. In future, it will be treated as `np.float64 == np.dtype(float).type`.
from ._conv import register_converters as _register_converters
[4]:
input_file_mask = 'data/mESC/perturbed-mesc-tpm-linear'
Read data¶
Here we have label, so we load both. However, the label is not used until evaluation.
[5]:
def preprocess(input_file_mask):
"""
Read in data and perform log transform (log2(x+1)), centering (mean = 1) and scaling (sd = 1).
"""
tpm = writer.read_df_from_binary(input_file_mask)
sttpm = pd.DataFrame(data=skl.preprocessing.scale(np.log2(tpm.values + 1)), index=tpm.index, columns=tpm.columns)
label = pd.read_csv(input_file_mask + '-label.csv', sep="\t", index_col=0)
return sttpm, label
sttpm, label = preprocess(input_file_mask)
There is no convention whether cells should be columns or rows. Here we require cells to be rows.
[6]:
sttpm.head()
[6]:
Gnai3 | Pbsn | Cdc45 | H19 | Scml2 | Apoh | Narf | Cav2 | Klf6 | Scmh1 | ... | RP23-345J21.2 | AC121960.1 | AC136147.1 | AC122013.1 | AC132389.1 | Gm11392 | AC160109.2 | AC154675.1 | AC156980.1 | RP23-429I18.1 | |
---|---|---|---|---|---|---|---|---|---|---|---|---|---|---|---|---|---|---|---|---|---|
G1_cell1_count | -0.240365 | -0.059976 | 0.077328 | 5.298087 | -0.714166 | 0.0 | -0.707230 | -0.059976 | -1.114342 | -0.368917 | ... | -0.149121 | 0.0 | -0.080859 | -0.381839 | -0.846925 | -0.059976 | -0.081147 | 0.0 | -0.147211 | 0.049833 |
G1_cell2_count | -0.368984 | -0.059976 | 0.615395 | -0.168451 | -0.842523 | 0.0 | 0.337309 | -0.059976 | 1.437104 | 0.184563 | ... | -0.149121 | 0.0 | -0.080859 | -0.381839 | -0.846925 | -0.059976 | -0.081147 | 0.0 | -0.147211 | -1.326395 |
G1_cell3_count | -1.588597 | -0.059976 | -1.439700 | -0.168451 | -0.842523 | 0.0 | -0.707230 | -0.059976 | -1.337381 | -0.672972 | ... | 2.548733 | 0.0 | -0.080859 | -0.381839 | -0.616570 | -0.059976 | -0.081147 | 0.0 | -0.147211 | -1.326395 |
G1_cell4_count | -2.043812 | -0.059976 | 0.766249 | -0.168451 | -0.842523 | 0.0 | 0.873861 | -0.059976 | 1.408474 | -0.672972 | ... | -0.149121 | 0.0 | -0.080859 | -0.381839 | 2.898387 | -0.059976 | -0.081147 | 0.0 | -0.147211 | -1.326395 |
G1_cell5_count | -1.668098 | -0.059976 | 0.182776 | -0.168451 | -0.842523 | 0.0 | -0.707230 | -0.059976 | -0.869770 | -0.672972 | ... | -0.149121 | 0.0 | -0.080859 | -0.381839 | -0.846925 | -0.059976 | -0.081147 | 0.0 | -0.147211 | -0.154229 |
5 rows × 38293 columns
[7]:
label.head()
[7]:
stage | subcluster | |
---|---|---|
G1_cell1_count | g0/g1 | perturbed |
G1_cell2_count | g0/g1 | intact |
G1_cell3_count | g0/g1 | intact |
G1_cell4_count | g0/g1 | intact |
G1_cell5_count | g0/g1 | perturbed |
Set up the model and fit the model¶
Fitting the model may take some time. Using a GTX 960M GPU it takes 6 minutes.
[8]:
model = cyclum.PreloadCyclum(sttpm.values, q_circular=3, q_linear=1)
[9]:
pseudotime, rotation = model.fit()
pretrain burnin
step 2000: loss [0.17363924, 38648384.0, 143098.72] time 9.98
pretrain train
step 2000: loss [0.17294206, 38648396.0, 143098.72] time 7.21
step 4000: loss [0.17294218, 38648390.0, 143098.72] time 6.14
midtrain burnin
step 2000: loss [0.17294218, 13706.275, 66501.27] time 30.63
midtrain train
step 2000: loss [0.17294218, 13703.313, 66501.27] time 30.84
step 4000: loss [0.17294218, 13703.31, 66501.27] time 28.50
finaltrain train
step 2000: loss [0.25978315, 13456.805, 36317.523] time 41.18
step 4000: loss [0.3560579, 13454.46, 19613.684] time 37.69
step 6000: loss [0.40473315, 13453.682, 10077.552] time 37.72
finaltrain refine
step 2000: loss [0.41543347, 13453.116, 8114.8086] time 41.51
step 4000: loss [0.43487915, 13452.949, 6591.534] time 37.74
step 6000: loss [0.44793454, 13452.951, 5367.22] time 37.72
step 8000: loss [0.45629007, 13452.896, 4374.1514] time 37.78
step 10000: loss [0.45684564, 13452.864, 3562.853] time 37.73
Full time 422.85
Illustrations¶
We illustrate the results on a circle, to show its circular nature. There is virtually no start and end of the circle. Red, green and blue represents G0/G1, S and G2/M phase respectively. The inner lines represents single cells. The cells spread across the The areas outside
[10]:
%aimport cyclum.illustration
[11]:
color_map = {'stage': {"g0/g1": "red", "s": "green", "g2/m": "blue"},
'subcluster': {"intact": "cyan", "perturbed": "violet"}}
cyclum.illustration.plot_round_distr_color(pseudotime, label['stage'], color_map['stage'])
[11]:
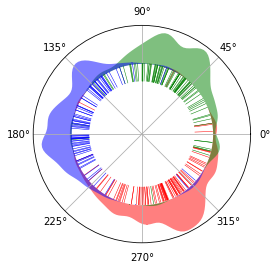
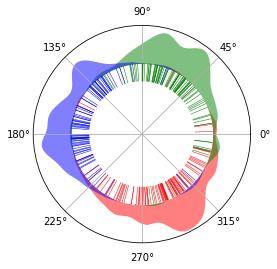
[ ]:
Subclone analysis¶
Many analysis are done in R, so we also choose R to do this job. On the jupyter/python side, package rpy2 is needed to run R inline. On the R side, packages Rtsne and matrixStats are required. It takes some time to transfer data from python to R.
The data corrected by cyclum shows better separation of two subclones in the tsne plot.
[12]:
sttpm2 = sttpm - np.concatenate([np.cos(pseudotime + i * 2 * np.pi / 3) for i in range(3)], axis=1) @ rotation
[13]:
%load_ext rpy2.ipython
[38]:
%%R
library(Rtsne)
library(matrixStats)
library(mclust)
calc_class_score_cv <- function(x, cls){
cv = cvMclustDA(MclustDA(x, cls, G=1, verbose = F), verbose = F)
return(1 - cv$error)
}
[14]:
%R -i label
%R -i sttpm sttpm = as.matrix(sttpm)
%R -i sttpm2 sttpm2 = as.matrix(sttpm2)
%R print(dim(sttpm))
/home/shaoheng/.conda/envs/tensorflow-gpu/lib/python3.6/site-packages/rpy2/robjects/pandas2ri.py:190: FutureWarning: from_items is deprecated. Please use DataFrame.from_dict(dict(items), ...) instead. DataFrame.from_dict(OrderedDict(items)) may be used to preserve the key order.
res = PandasDataFrame.from_items(items)
[1] 279 38293
[48]:
%%R
set.seed(2019)
nonzero_mask <- colSds(sttpm) > 1e-3
original_tsne <- Rtsne(sttpm[, nonzero_mask], pca_scale = TRUE, theta=0.1)
plot(original_tsne$Y, col=as.factor(label$subcluster), pch=19)
title(paste('original data separation rate:', calc_class_score_cv(original_tsne$Y, label$subcluster)))
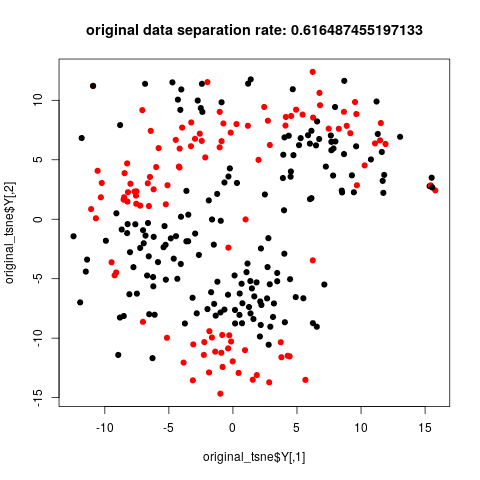
[49]:
%%R
nonzero_mask <- colSds(sttpm2) > 1e-3
corrected_tsne <- Rtsne(sttpm2[, nonzero_mask], pca_scale = TRUE, theta=0.1)
plot(corrected_tsne$Y, col=as.factor(label$subcluster), pch=19)
title(paste('corrected data separation rate:', calc_class_score_cv(corrected_tsne$Y, label$subcluster)))
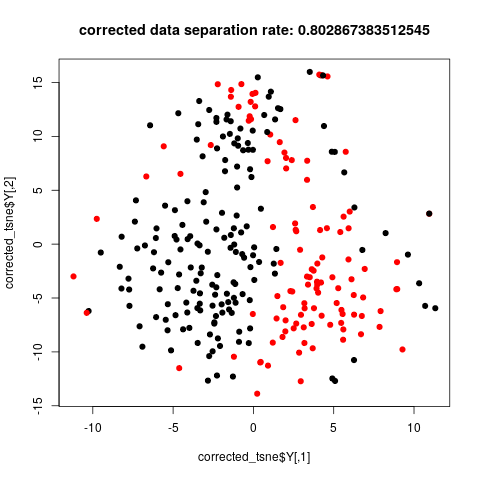